In a latest research printed in The Lancet Regional Well being-Southeast Asia, researchers developed a synthetic intelligence (AI)-based predictive system (AIPS) mannequin for the early detection of lung most cancers by combining radiological, scientific, and genetic information.
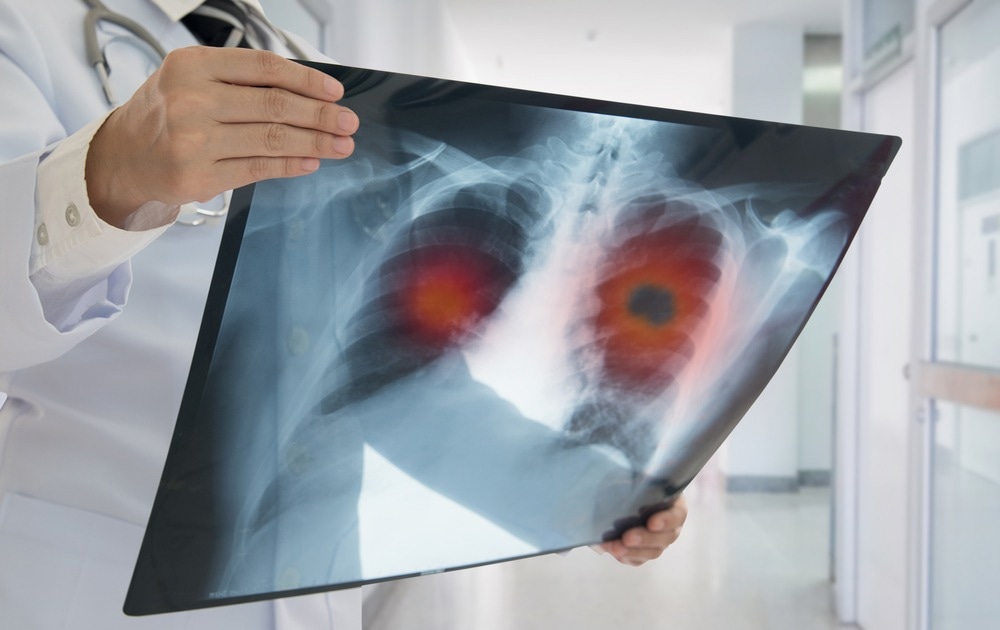
Background
Lung most cancers prognosis has improved on account of molecular targets and focused remedies, together with remedy for somatic epidermal progress issue receptor (EGFR) mutations. Nonetheless, next-generation sequencing (NGS) is unavailable in resource-constrained nations like India. An AI-based pipeline is required to acknowledge lung nodule traits in computed tomography (CT) scans and predict EGFR mutation likelihood, permitting for near-optimal care and remedy.
Concerning the research
Within the current research, researchers demonstrated the APIS pipeline for figuring out and predicting the presence of EGFR-mutant lung nodules from CT scans in resource-constrained conditions resembling India.
Utilizing EGFR sequencing and CT imaging information from 2,277 lung most cancers sufferers, the researchers created an automatic lung nodule identification and characterization algorithm. They targeting Indians (Cohorts 1, 2, and three), opposite to prior analysis, which primarily centered on people of white and Chinese language descent.
The group collected information on the Indian inhabitants from the Rajiv Gandhi Most cancers Institute and Analysis Centre, India, and the white inhabitants (Cohort 4) information from The Most cancers Imaging Archive (TCIA). They created the AI prediction system-Nodule mannequin (AIPS-N) by acquiring the Lung Picture Database Consortium picture assortment (LIDC-IDRI) computed tomography imaging information (Cohort 5), which included 1,010 sufferers (denoted by 244,527 photos).
The researchers pre-processed CT scans with windowing strategies to enhance lung visibility, then interpreted image annotations and used computerized lung segmentations to detect and segregate the lung area within the picture. They skilled the sooner region-based convolutional neural community (R-CNN) mannequin with the parsed picture annotations and the corresponding pre-processed photos.
The group preprocessed LIDC-IDRI photos to evaluate the anatomy and determine pathologies. They normalized the DICOM depth values to a specified vary earlier than utilizing windowing strategies to attain a constant depth vary throughout photos from varied cohorts. They handed the coaching dataset, which included masks, picture annotations, and slices, into the pre-trained primary mannequin, which extracted options from enter photos and generated high-level multi-scale convolutional-type function maps.
The researchers used the Area Proposal Community (RPN) on multiple-scale convolutional function maps to anticipate area proposals and possible areas within the image which will embrace gadgets of curiosity. Anchor deltas had been prefabricated bounding bins with diversified side ratios and sizes positioned onto the convolutional function maps to assist enhance the anchor bins and align them extra intently with picture objects.
The AIPS-Nodule mannequin used a yellow field to visually emphasize and enclose the lung area of curiosity in CT scans, permitting for extra investigation. The automated pulmonary segmentation and object identification fashions had been constructed individually for every nodule function, yielding 5 AIPS-N function fashions. The researchers used primary fashions such because the ResNet101-Characteristic Pyramid Community (R101-FPN) and Sooner region-based CNN to extract picture options and prepare the mannequin. They used 70%, 15%, and 15% of the information for mannequin coaching, validation, and testing, respectively.
Outcomes
The deep studying mannequin might substitute machine studying (ML) fashions by including AIPS-N outcomes, which enhanced ML efficiency. The AIPS-Nodule mannequin might robotically detect and characterize lung nodules, with a imply AP50 rating of 70%. The AIPS-M system mixed the AIPS-Nodule outcomes with scientific parameters to foretell EGFR genotype to yield space beneath the receiver-operating attribute curve (AUC) values ranging between 0.6 and 0.9.
Cohort 1-trained ML algorithms had a imply AUC of 0.85 within the validation subgroup. Random Forest, Randomized Search Cross-Validation, XGBoost, and AIPS-Mutation ML fashions skilled on the Indian inhabitants and white cohort produced a imply AUC of 0.8 when examined on Cohort 4. In testing Cohort 4, the imply AUC of the ML fashions skilled utilizing the primary cohort elevated from 0.6 to 0.9, demonstrating the optimistic affect of integrating AIPS-N rankings on predictive capabilities.
The analysis included an Indian male smoker aged 71 years recognized with squamous cell carcinoma and carrying the EGFR gene mutation. The AIPS-Nodule mannequin precisely recognized the options and site of the detected nodule, assigning spiculation and sphericity to class 1. AIPS-Mutation fashions skilled on Cohort 1 had been used to find out the EGFR gene standing of the male affected person, who was clinically decided as mutated. All six machine studying algorithms predicted EGFR standing as mutated, yielding a ‘True Constructive’ end result.
The research confirmed that CT imaging paired with AIPS automated pulmonary nodule evaluation may predict EGFR gene presence and determine people with EGFR mutations cost-effectively and non-invasively.
The AIPS-Nodule mannequin might detect and describe lung nodules, whereas the AIPS-Mutation mannequin predicted EGFR genotype by integrating AIPS-N findings with scientific variables. The research’s findings may assist oncologists prioritize sufferers for tailor-made medicines, enhance affected person care, and improve world healthcare requirements.
The progressive AIPS method may benefit resource-limited conditions and add to earlier proof by broadening the scope of nodule investigation to incorporate thorough characterization and affiliation with the EGFR mutation.
Supply hyperlink