Well being entails the wellbeing of the bodily, emotional, psychological, and mental domains of man. These are deeply impacted by social elements, typically termed the social determinants of well being (SDoH). Nevertheless, these should not documented clearly or adequately in digital well being data (EHRs).
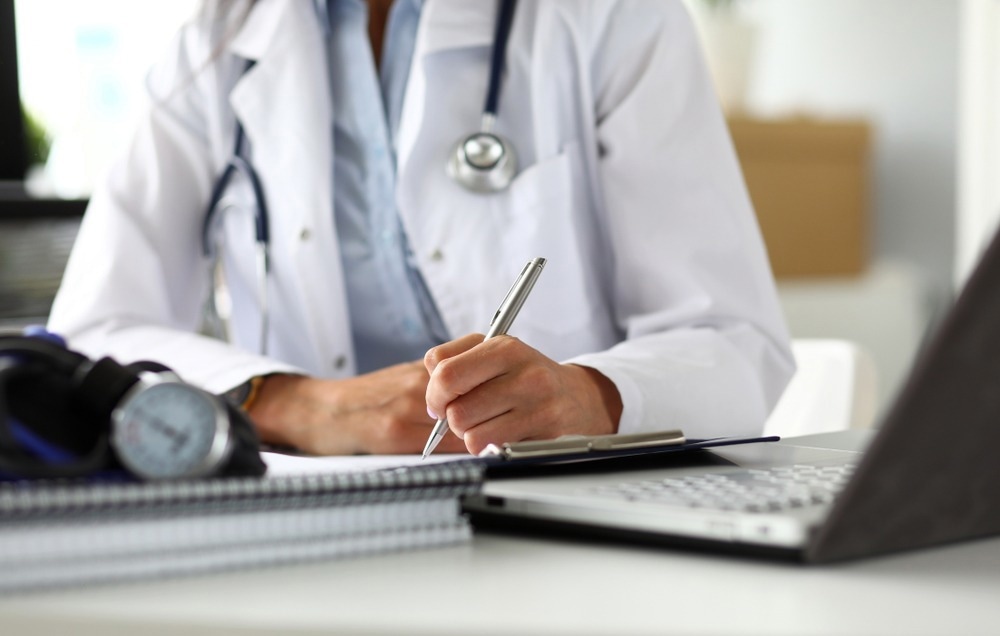
A brand new research in npj Digital Drugs explores using giant language fashions (LLMs) to acquire such important information from EHRs to enhance analysis outcomes and supply higher medical care.
Background
The significance of SDoH lies of their documented means to contribute to well being disparities. They rely upon the person’s means to spend on and entry health-promoting life and high-quality medical amenities by way of wealth, energy, and assets. Apart from this direct affect, antagonistic SDoH not directly contributes to neural and endocrine alterations and low-level irritation which will result in bodily and psychological ill-health.
“SDoH are estimated to account for 80–90% of modifiable elements impacting well being outcomes.”
Regardless of this significant place, they’re not often captured in a scientific or complete method in EHRs and, due to this fact, go with out intervention. It’s essential to shift the documentation of those elements from the free textual content of clinic notes to the structured format of EHRs to select sufferers who is likely to be helped by way of social work or by supplying needed assets.
Computational advances like pure language processing (NLP) may also help switch this free textual content to formatted information for medical analysis, however the efficiency of those instruments stays unmeasured.
Furthermore, the event of high-quality giant language fashions (LLMs) mandates their analysis for contributing extra information by mining EHRs, and the identification of one of the best methods to generate and use this information.
These superior fashions may additionally produce such information for additional processing by smaller LMs. Furthermore, the potential for bias must be understood earlier than it may be used for analysis.
The present research examines varied strategies for the extraction of SDoH by LLMs, specializing in six essential elements. The six lessons utilized by the LLMs on this research included employment, housing, Transportation, parental standing, relationship, and social assist.
It additionally explores the utility of including these artificial information whereas fine-tuning the fashions. Lastly, it in contrast varied LLMs for efficiency in figuring out SDoH and the possibilities of introducing bias into the predictions.
What did the research present?
The researchers discovered that among the many fashions used; specifically, BERT, and varied Flan-T5 fashions, and the ChatGPT-family, the fashions that carried out greatest to extract any point out of SDoH have been fine-tuned Flan-T5 XL, which excelled in 3 of the 6 classes with artificial information. In the meantime, for antagonistic mentions of SDoH, it was Flan-T5 XXL with out artificial information.
The fewest parameters have been tuned for each these fashions. The bigger the mannequin, the higher the efficiency.
When the artificial information extracted and processed by LLMs have been included into the coaching datasets, the outcomes differed by fashions and with the structure of the code. The biggest enchancment occurred when the coaching dataset had the smallest variety of situations and when the gold-only educated mannequin carried out the worst. Total, nonetheless, there was an enchancment within the efficiency with smaller fashions.
When gold information was progressively eliminated, efficiency remained in line with artificial information addition till about 50% had been eliminated. Conversely, with out artificial information, it started to fall after 10-20% of gold information was eliminated, as can be the case in a low-resource setting.
When in comparison with ChatGPT, the fine-tuned Flan-T5 fashions did higher than both GPT-turbo-0613 and GPT4–0613 on Any SDoH job however much less effectively on Hostile SDoH job. The very best-performing fine-tuned fashions produced higher outcomes when set to zero- or few-shot settings. The exception was when GPT was set to 10-shot prompting for antagonistic SDoH.
The fine-tuned fashions have been additionally extra constant of their predictions after incorporating SDoH elements like race and gender, indicating that their algorithms have been much less biased. That’s, ChatGPT was more likely to change its classification when feminine gender was assigned for Any SDoH job as a substitute of the male gender.
Equally, gold-labeled Assist class information for each Any and Hostile SDoH duties generated the best threat of producing discrepancies within the predictions when ChatGPT was used, at 56% and 21%, respectively. The identical sort of knowledge for Employment class injected the best possibilities for discrepant prediction for Any SDoH job with the fine-tuned mannequin vs Transportation for Hostile SDoH job, at 14% and 12%, respectively.
Lastly, these fashions captured nearly 94% of sufferers with antagonistic SDoH, in comparison with 2% with customary EHR follow, that’s, ICD-10 codes. This covers a really giant hole of 92%.
The researchers have been thus in a position to develop fashions that labeled sufferers by six SDoH classes utilizing medical notes. They detected the variations in efficiency between the extra generally used BERT classifier in comparison with the LLMs resembling Flan-T5 XL and XXL.
After fine-tuning, the fashions carried out higher than ChatGPT and resisted deterioration following the introduction of artificial demographic descriptive phrases.
What are the implications?
All fashions have been in a position to establish free-text sentences with out overt SDoH mentions, although Parental Standing mentions carried out the worst for Any SDoH mentions, together with Transportation. For Hostile SDoH duties, the worst efficiency was for Parental Standing and Social Assist.
The superior efficiency of those fashions is spectacular given the truth that solely 3% of all sentences within the coaching set talked about any SDoH and that such descriptions are complicated in that means and language use. The findings of this research underlined earlier stories that one of the best efficiency in SDoH extraction used the whole medical file relatively than simply the part on Social Historical past since such information is commonly scattered all through the notes. Conversely, many varieties of notes fail to say Social Historical past.
The least talked about class was housing, however the highest performing mannequin did effectively at classifying this issue, suggesting the usefulness of LLMs in augmenting information assortment in real-world conditions the place the data could be very scantily reported and therefore most simply missed when manually compiled.
Furthermore, the present analysis might assist resolve the issue of amassing information in sparsely documented classes from the big quantity of textual content in EHRs. The ChatGPT fashions GPT3.5 and GPT4 have been additionally discovered to be precious for such duties, doubtlessly pending additional research.
The features from utilizing LLMs to establish SDoH in relation to medical historical past are at the very least two-fold: “bettering real-world proof on SDoH and helping in figuring out sufferers who may gain advantage from useful resource assist.” This work additionally highlights the necessity to embrace these elements when predicting well being outcomes.
Supply hyperlink
Fantastic site A lot of helpful info here Im sending it to some buddies ans additionally sharing in delicious And naturally thanks on your sweat